We are often asked how much more accurate will weather forecasts be in the future. Weather prediction skill has extended by about a day-per-decade over the last 30 years, mainly due to greatly improved data assimilation schemes, super computers and more advanced computer simulations of the weather. Despite these efforts it still remains only an approximation of the actual conditions and our numerical weather models are at best a sophisticated approximation of reality. The slightest discrepancy in the initial approximations means that over time a weather forecast will diverge from the actual weather.
So there is a limit on how far in to the future we can predict the day to day weather as these computer simulations need to conquer chaos theory where small changes completely transform the future of a system. It emerges in a prediction when a small unknown or error is amplified to the point where the prediction is destroyed. This is the reason why it is inherently difficult to make longer term predictions in chaotic systems such as the solar system, ecosystems, cultures, economies, politics, climate and the weather.
Our atmosphere and oceans are chaotic systems in continual motion with multiple chains of cause and effect whereby a small cause can eventually be amplified to greater and greater effects. Another important principle of chaos theory is unpredictability. At the smallest level it is impossible to know exactly where an atom is and how fast it is moving. It follows that the tiniest perturbation in the air can dramatically alter what happens next and so there will be gaps and uncertainties which amplify over time and eventually destroy the prediction.
This phenomenon of ultra sensitive dependence on initial conditions is commonly referred to as the “butterfly effect”, where something small at the beginning leads to something major in the end. Basically, because we can never assimilate the atmosphere 100% at the beginning (for model initialisation) then the ultimate outcome of an event is never truly known.
In broad terms, the current accuracy of a 7-day (144 hrs) forecast is similar to that of a 1-day (24 hrs) forecasts from 50 years ago. Skill then decreases out to 14-15 days after which there is little or no skill and things become random. One example of this trend in accuracy is the dramatic reduction of tropical cyclone (TC) track forecast errors over the last 25 years. The NHC reports that in the North Atlantic, the mean 72hr track forecast error decreased from 248nm in 1995 to 100nm in 2018. Current 72hr track forecasts have similar errors to 48hr forecasts from the mid-2000s and 24hr forecasts from the mid-1990s.
These improvements were driven by higher model resolution, improved data assimilation, better physics, and dramatic improvements in quality and quantity of observations (mainly from satellites) used to initialise the models. However, there is evidence that the forecast skill has levelled off over recent years in the North Atlantic, suggesting that operational forecasts may be approaching the limit of TC track predictability – see these latest NHC Official Track Skills and Trend images below which include data from the 2019 Hurricane season.
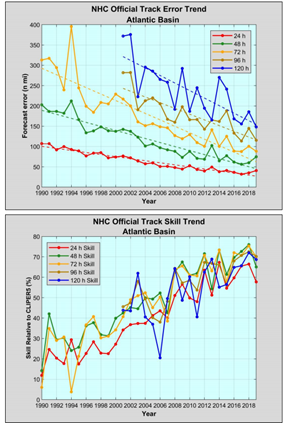
So what is chaos theory? Chaos theory was discovered by Edward Lorenz in 1961 when he ran a simple weather deterministic model simulating two months of weather. He later rerun the simulation but discovered vastly different results, and on investigation discovered he had made a one-part-in-a-thousand rounding error compared to the first run when he re-entered the data. This tiny error had huge significance. His experiment revealed that the slightest difference in initial conditions will grow and eventually produce widely divergent results.
Chaotic systems are predictable for a while and then ‘appear’ to become random. For weather forecasting, the uncertainty in a forecast therefore increases exponentially with time. The amount of time that the behaviour of a chaotic system can be effectively predicted depends on three things: how much uncertainty can be tolerated in the forecast, how accurately its current (or initialisation) state can be measured, and the system’s Lyapunov time; the associated chaotic system time limit for prediction accuracy known as the predictability horizon. Anything beyond this time is not much better than a random guess.
Currently, weather forecasts up to 8-10 days are considered reliable. Thereafter skill fades such that there is little or no skill by day 15. The latest evidence suggests that Lyapunov time for weather forecasting is currently around 15 days. By comparison the evolution of the solar system is chaotic with a Lyapunov time of 4-5m years. In looking ahead, there is significant demand for development of numerical weather prediction systems via continual improvements to the observing, modelling and data assimilation systems. This effort will likely extend the predictability horizon and the one-day-per-decade improvement could be maintained for the next 20-25 years.
So what does this mean for ship weather and route optimisation? Ship schedules, reliability, fuel consumption and emissions are very weather dependent. One key reason is the stochastic (random) behaviour of metocean processes that generates uncertainty in weather and ocean forecasts. Watch out for the next blog in this series on how we are addressing these issues.
Stay connected and safe.